Leo Klarner
PhD Student in AI for Drug Discovery at the University of Oxford
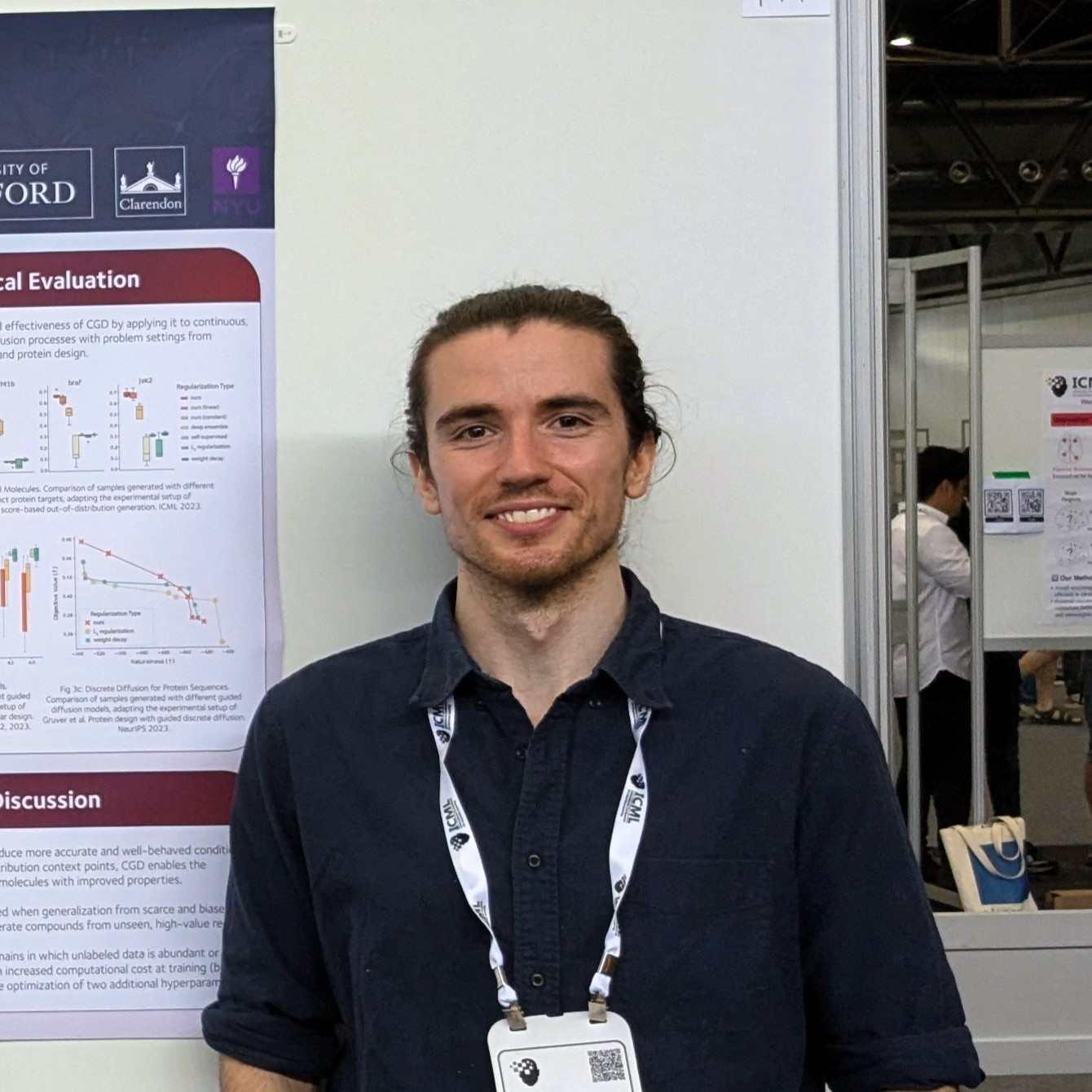
leo.klarner [at] stats.ox.ac.uk
I’m a final-year PhD student in the Oxford Protein Informatics (OPIG) and CompStats & Machine Learning (OxCSML) groups. My research focuses on developing more robust and data-efficient generative models for applications in early-stage drug discovery and protein design. Specifically, I’m working on improving out-of-distribution generalisation (ICML 2023, ICML 2024) and generative modelling under domain-informed constraints (TMLR, 2023, NeurIPS 2023).
This work requires familiarity with both modern deep learning and the realities of practical drug discovery, which I have been fortunate to pick up from my supervisors Yee Whye Teh (Oxford/DeepMind), Charlotte Deane (Oxford) and Garrett Morris (Oxford), as well as Torsten Schindler and Michael Reutlinger (Roche). My work is funded by a Clarendon Scholarship (Oxford’s flagship academic merit scholarship for graduate students) and additional partnership awards from Brasenose College, Oxford and Roche.
I feel strongly about understanding the data I work with and have spent many weeks in ChEMBL, PubChem and the PDB. I am also interested in curating more realistic datasets and benchmarks (ICML 2023, AI for Chemistry Best Poster Award) and am a main contributor of the open-source Gauche package (NeurIPS 2023).
Before starting my PhD, I completed a BSc. in Interdisciplinary Sciences (chemistry, biology and CS) at ETH Zürich. During this time, I had the opportunity to design and synthesise antimicrobial peptides with Prof Gisbert Schneider and engineer bacteria for targeted cancer therapy with Prof Simone Schürle-Finke.